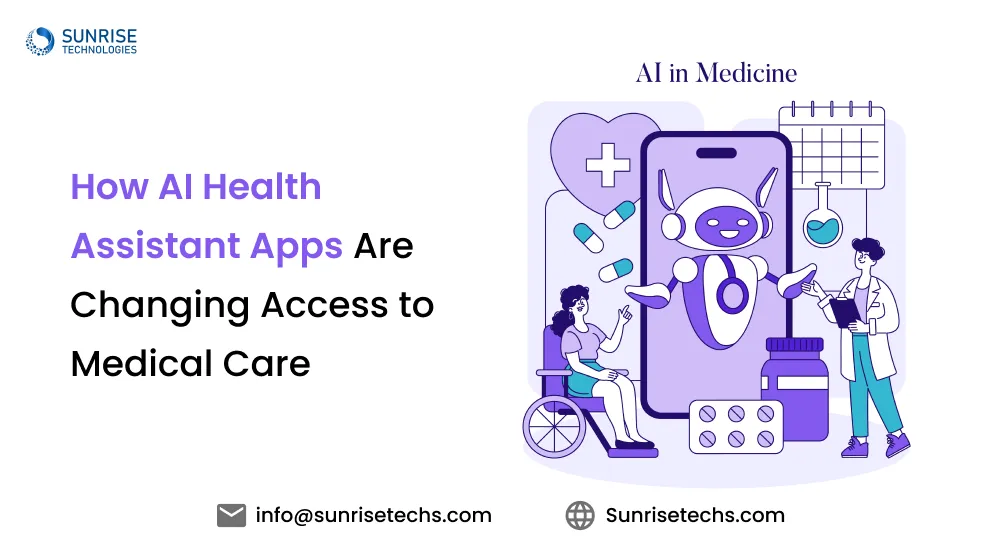
How AI Health Assistant Apps Are Changing Access to Medical Care
May 22, 2025
In recent years, AI health assistant apps have taken center stage in transforming how we access medical care, becoming the backbone of accessible, efficient, and intelligent healthcare delivery.
- According to a recent report, AI-powered healthcare solutions have the potential to save up to $150 billion annually for the U.S. healthcare system by 2026.
- In fact, 63% of healthcare consumers are already comfortable using AI-enabled medical apps to manage their health.
- The global market for AI health assistant apps is projected to grow at a CAGR of 38.1% between 2024 and 2030, reflecting the accelerating shift toward AI-assisted healthcare ecosystems
This growing trust and reliance on digital health assistants are reshaping the landscape of care delivery, particularly in remote and underserved communities where access to traditional healthcare is often constrained.
Empowering Hospitals, Clinics, and Medical Departments:
To keep pace with this digital evolution, hospitals, medical departments, and healthcare providers can integrate AI-assisted healthcare apps into their operations to:
- Automate routine tasks like appointment scheduling and follow-ups
- Enable smart triage systems for quicker patient assessment
- Improve AI-driven patient engagement through 24/7 virtual health consultations
- Enhance decision-making with predictive analytics and personalized treatment recommendations.
This blog explores how AI health assistant apps are revolutionizing medical care,, examining their evolution, functionality, real-world impact, and the very answer to how can AI health assistants improve healthcare accessibility?
Evolution of AI in Healthcare Over the Ages
Let’s take a step back and look at how far we’ve come in the world of AI in healthcare.
It all started in the 1970s, when early expert systems like MYCIN were developed to assist doctors in diagnosing bacterial infections. These systems were rule-based, meaning they followed predefined logic rather than learning from data. That was just the beginning.
Today, we live in the era of AI-powered health assistant apps, offering real-time medical support, predictive analytics, and even personalized treatment plans, all from the convenience of your smartphone.
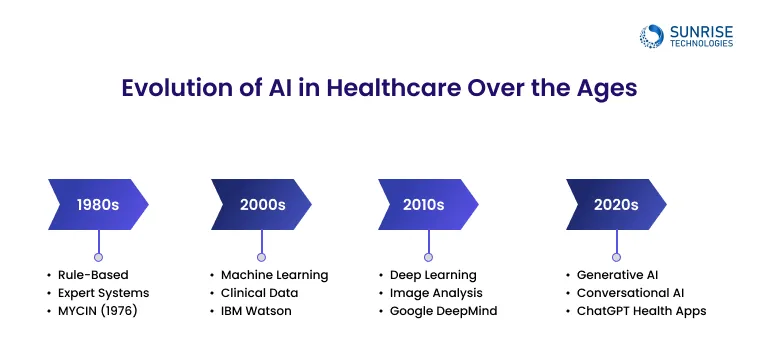
- 1950s–1980s: Early expert systems like MYCIN (1976) used rule-based algorithms to diagnose bacterial infections.
- 1990s–2000s: Machine learning (ML) entered diagnostics, with IBM’s Watson analyzing clinical data.
- 2010s: Deep learning revolutionized image analysis (e.g., Google’s DeepMind detecting diabetic retinopathy).
- 2020s: Generative AI (e.g., ChatGPT) now powers AI-powered health assistants that simulate human-like conversations.
So, how did this evolution happen? What powered this shift from simple diagnostic tools to intelligent, always-on health assistants?
Key Drivers Behind the Evolution:
Advancements in machine learning and neural networks: Modern AI health assistant apps rely on deep learning models trained on massive datasets to detect symptoms, predict illnesses, and even suggest medications.
Big data and electronic health records (EHRs): The integration of patient data from EHRs has enabled AI-powered healthcare solutions to deliver more accurate and personalized recommendations.
Natural Language Processing (NLP): With NLP, these apps can engage in smooth, conversational interactions, transforming into digital health assistants that understand and respond like a human doctor.
What Is AI in Healthcare and How AI Is Changing Healthcare
In simple terms, AI in healthcare refers to the use of artificial intelligence technologies, such as machine learning, computer vision, and natural language processing (NLP) to automate, optimize, and support both clinical and administrative workflows.
So, before reading the blog, let’s be clear on one thing, What is an AI health assistant app? Today, AI health assistant apps go far beyond answering basic questions. They’re transforming care delivery through:
- Best AI-powered health assistant for medical consultations that offer instant medical advice
- Predictive algorithms that support early disease detection
- Wearable integrations to monitor vitals and trigger real-time alerts
- Data-driven decision support tools that help physicians act faster and smarter
From AI-powered medical apps for underserved communities to AI-powered virtual assistants for hospitals, these solutions are closing critical gaps in healthcare access, reducing delays, and enhancing patient outcomes at scale.
Use Case and Applications of AI in Healthcare
The scope of Artificial Intelligence in healthcare goes far beyond virtual consultations and symptom checkers. In fact, some of the most groundbreaking innovations are happening behind the scenes, powering diagnostics, accelerating research, and shaping public health policies. Let’s dive into the advanced AI-driven healthcare applications in modern healthcare that are transforming the industry from the inside out.
One of the most impactful areas of AI in healthcare is in medical image analysis. From X-rays to MRIs, AI algorithms are trained to spot patterns the human eye might miss. AI in medical image analysis is transforming diagnostic accuracy by leveraging convolutional neural networks (CNNs) and computer vision techniques to analyze radiological scans.
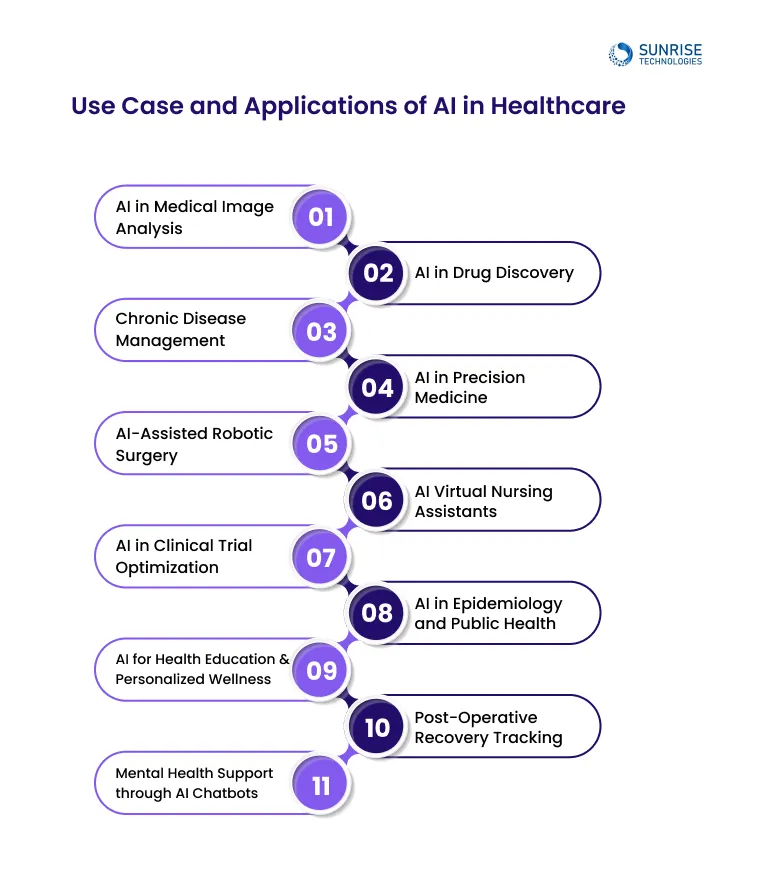
- Deep learning models detect anomalies like tumors, fractures, and infections
- Faster and more accurate second opinions in radiology and pathology
- Integration of DICOM image processing with AI improves radiologist workflows
- Improved early detection for diseases like cancer and neurological disorders
Developing a new drug is time-consuming and expensive, but AI is changing that. AI-powered drug discovery platforms analyze millions of compounds to identify potential candidates, and even suggest new therapeutic uses for existing drugs. AI in drug discovery and repurposing accelerates the process by combining generative models like GANs and reinforcement learning with molecular simulations.
- Predictive modeling for identifying promising molecules
- Accelerated compound screening using AI simulations
- QSAR (Quantitative Structure-Activity Relationship) modeling to screen candidate compounds
- NLP-based mining of biomedical literature for drug repurposing opportunities
- Discovering new indications for FDA-approved drugs
So, How AI health assistant apps are transforming chronic disease management? AI enables continuous monitoring and predictive analytics for managing chronic illnesses, enhancing early intervention and personalized care strategies. These systems leverage real-time data to support clinical decision-making and optimize long-term treatment plans.
- Forecasts disease progression using historical and real-time health data.
- Uses wearables and IoT devices to track vitals like blood glucose and heart rate.
- AI algorithms tailor medication and therapy based on individual patient profiles.
- Notifies providers of anomalies or risk patterns to prevent critical events.
- Seamlessly syncs patient data across platforms for holistic care coordination.
No two patients are the same, so why should their treatment be? AI in precision medicine helps analyze genetic, lifestyle, and environmental data to craft personalized treatment plans.
- Genomic data analysis for targeted cancer therapies
- AI-driven risk predictions based on patient history
- AI models interpret genomics, proteomics, and metabolomics to tailor treatments
- Use of Bayesian networks and ensemble learning for risk stratification
- Custom medication recommendations based on biomarkers
Surgical robots are becoming smarter, thanks to AI.
With AI-assisted robotic surgery, surgeons gain enhanced control, reduced error margins, and better outcomes, especially in complex or minimally invasive procedures.
- AI-powered motion scaling and tremor reduction
- Real-time feedback during surgeries via computer vision
- Advanced motion scaling algorithms reduce tremors in delicate procedures
- Integration of AI-powered vision systems for tissue recognition and mapping
- Shorter recovery times and improved precision
Patients don’t always have access to nurses round-the-clock, but now they have virtual ones.
AI-powered virtual nursing assistants offer constant support, from medication reminders to post-discharge guidance and emotional reassurance.
- 24/7 patient Q&A using natural language processing (NLP)
- Integration with FHIR (Fast Healthcare Interoperability Resources) for EHR access
- Daily wellness check-ins and treatment reminders
- Early detection of deteriorating conditions
Clinical trials can be notoriously slow and inefficient. AI in clinical trial optimization streamlines everything, from patient recruitment to predictive analytics that improve study outcomes.
- AI models identify ideal trial candidates faster
- Predict dropout risks and optimize protocol design
- Clustering algorithms for identifying ideal patient cohorts
- Digital twin modeling to simulate trial scenarios and outcomes
- Improve data quality and reduce trial durations
Tracking and predicting disease outbreaks used to take weeks. Now, AI can do it in real time.
AI in epidemiology and public health helps governments and health organizations act faster and smarter.
- Real-time outbreak prediction using big data and machine learning
- Pattern detection from public health records and social media
- Resource allocation and emergency response planning
- Digital twin modeling to simulate trial scenarios and outcomes
- Improve data quality and reduce trial durations
Education drives prevention, and AI makes it personalized. Digital health assistants use patient data and behavior patterns to deliver custom wellness tips, daily habits, and preventive healthcare advice tailored to each user.
- Daily fitness and nutrition reminders
- Preventive health screening suggestions
- Behavioral pattern recognition using recurrent neural networks (RNNs)
- AI-curated wellness content based on age, gender, and history
After surgery, patients need consistent care and follow-up monitoring. AI-powered healthcare solutions help track recovery metrics, monitor symptoms, and provide timely recommendations for next steps.
- AI-generated recovery plans based on surgery type
- Monitoring of wound healing and pain levels
- Virtual check-ins through AI health assistant apps
After surgery, patients need consistent care and follow-up monitoring. AI-powered healthcare solutions help track recovery metrics, monitor symptoms, and provide timely recommendations for next steps.
- AI-generated recovery plans based on surgery type
- Monitoring of wound healing and pain levels
- Virtual check-ins through AI health assistant apps
Emotional well-being is just as important as physical health. AI-powered mental health chatbots use Natural Language Processing (NLP) to engage users in conversations, offer cognitive behavioral therapy (CBT) techniques, and provide instant emotional support, especially during off-hours.
- On-demand conversations for anxiety or depression support
- Guided CBT sessions via conversational interfaces
- Real-time access to CBT modules powered by AI-guided pathways
- Escalation to professionals when needed
From appointment scheduling to symptom checking, we develop scalable AI apps tailored to your organization’s healthcare goals.
Cost of AI-Assisted Healthcare Apps
When it comes to developing an AI-assisted healthcare app, costs can vary widely depending on the app’s complexity, integrated features, and the tech stack used. Whether you’re building a lightweight symptom checker or a full-scale virtual nursing platform, understanding the key cost drivers can help you plan better.
Here’s a quick breakdown of the estimated development cost ranges:
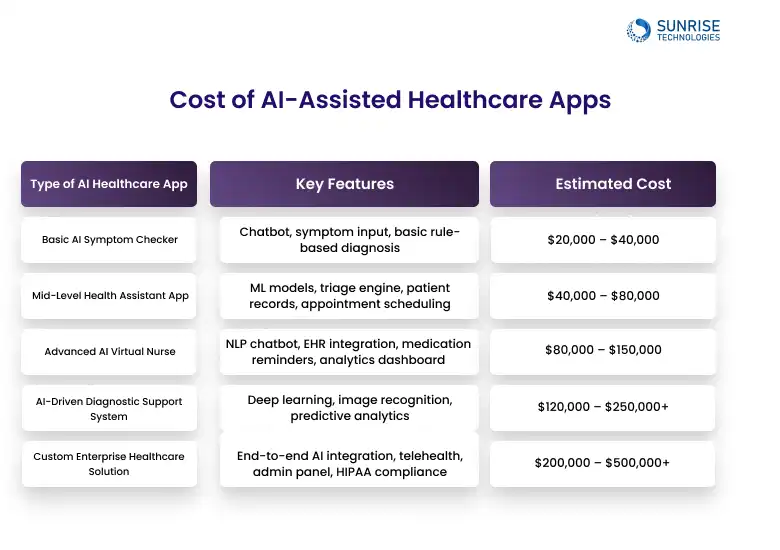
- 1. AI Model Complexity: Rule-based vs. machine learning vs. deep learning
- 2. Data Sources: Integration with EHR, SNOMED CT, ICD-10, or real-time IoT data
- 3. Compliance Needs: HIPAA (USA), GDPR (EU), and other data privacy regulations
- 4. Platform Type: Web, iOS, Android—or all three
- 5. Ongoing Maintenance: Continuous model training, app updates, and user support
Develop your own AI model that understands, predicts, and delivers results like never before.
How AI health assistant apps are transforming medical care
The traditional model of healthcare often meant long waits, limited consultation time, and barriers for those in remote or underserved areas. But not anymore. AI health assistant apps are revolutionizing access to medical care, delivering personalized, intelligent, and on-demand support, right from a smartphone.
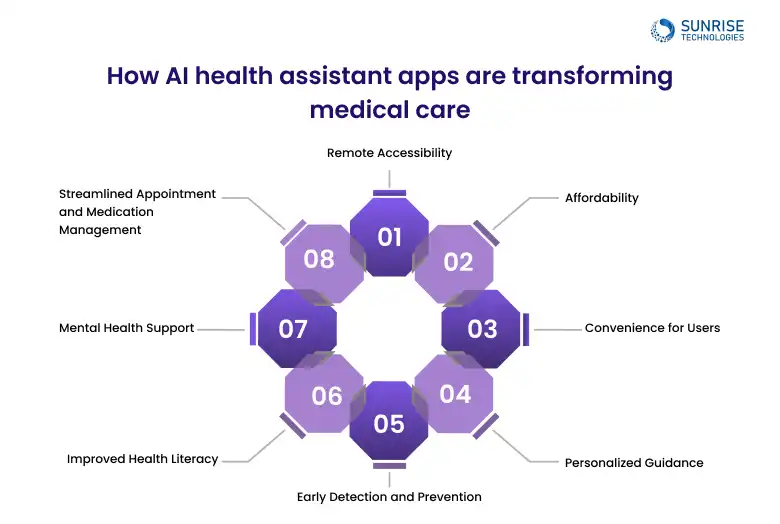
Let’s explore how these intelligent apps are bridging the healthcare divide and benefits of AI-powered health assistant apps in healthcare
AI apps are eliminating geographical barriers by bringing healthcare to your fingertips, regardless of your location, through AI in Remote Healthcare Technology.
- Ideal for people in rural or remote areas
- Supports patients with mobility challenges or chronic illnesses
- Leverages AI-driven triage systems and telehealth integrations
Healthcare should be a right, not a luxury,and AI is making it more affordable with the Best AI Apps for Low-Cost Medical Access
- Automates symptom checks, reducing the need for in-person visits
- Offers low-cost access to basic medical advice and education
- Reduces operational costs for healthcare providers
In today’s fast-paced world, convenience in healthcare is more important than ever.
- Provides 24/7 access to health guidance
- Helps users track symptoms and progress over time
- Reduces dependency on traditional appointment-based models
Every patient is unique, and AI health assistant apps are built to understand that.
- Leverages machine learning to understand user behavior and health history
- Offers tailored suggestions based on vitals, lifestyle, and pre-existing conditions
- Improves treatment adherence through personalized nudges
AI healthcare apps for remote patient monitoring enhance health data, and these apps become your digital early warning system.
- Detects anomalies in vital signs and behavior patterns
- Uses predictive analytics to anticipate potential risks
- Encourages users to seek preventive care before conditions escalate
Understanding your health shouldn’t feel like decoding jargon. AI makes it simple, through AI Health Education Tools
- Delivers easy-to-understand, verified health content
- Explains treatment options, dosages, and side effects in layman’s terms
- Boosts confidence in informed decision-making
Mental wellness is no longer sidelined, thanks to AI mental health support apps, through Virtual AI Counseling
- Provides guided exercises for stress and anxiety
- Delivers CBT-based conversation models for emotional relief
- Ensures privacy and 24/7 availability for support
Organizing your health journey becomes effortless with these smart tools.
- Schedules appointments with doctors or clinics automatically
- Sends reminders for medication, vaccinations, or follow-ups
- Integrates with EHRs to maintain continuity of care
Real-world examples of AI Health Assistant Apps
IBM Watson Assistant is a conversational AI platform designed for healthcare providers, insurers, and patients. It streamlines patient support, symptom triaging, and clinical workflow automation.
IBM’s Watson Assistant leverages its robust Watson NLP and Watson Discovery platforms, using deep learning and machine learning to interpret medical queries, offer symptom triage, and support clinical workflows. It’s built on IBM’s DeepQA architecture, enabling advanced reasoning based on large biomedical datasets. It integrates directly with Electronic Health Record (EHR) systems like Epic and Cerner, supporting HIPAA compliance and real-time access to patient history.
AI Model Used:
- Watson Natural Language Understanding (NLU)
- Transformer-based contextual models, fine-tuned on biomedical datasets
Ada Health offers an AI-driven symptom assessment app that acts like a digital health companion. Users input their symptoms via a conversational interface, and Ada provides possible conditions, severity insights, and suggested next steps. The system is clinically validated and used by millions globally, with strong partnerships across the healthcare ecosystem.
Ada’s reasoning engine is based on probabilistic and rule-based approaches, simulating how a doctor might narrow down diagnoses through questioning. The app uses structured medical ontologies, real-time user data, and feedback loops to fine-tune its accuracy. It cross-validates symptom patterns against a knowledge base of thousands of diseases, making the output medically explainable. The platform emphasizes user personalization, multilingual capability, and data privacy compliance (GDPR, HIPAA).
AI Models Used:
- Bayesian Network Model
- Ensemble Machine Learning Models
- Reinforcement Learning
- Natural Language Processing (NLP)
Amazon has integrated healthcare-focused voice assistant features via Alexa and supports intelligent health data structuring through HealthLake, their HIPAA-eligible service.
Amazon’s AI health capabilities combine HealthLake (a fully managed, HIPAA-eligible data lake for healthcare) with Alexa’s health skills, creating a voice-first AI health assistant experience. HealthLake applies Amazon Comprehend Medical, a domain-specific NLP service that extracts key medical insights, such as diagnosis, symptoms, and medications—from unstructured data like doctor’s notes. The system supports FHIR (Fast Healthcare Interoperability Resources) for seamless data exchange and includes predictive ML models for trend analysis. Alexa uses Amazon Lex, a deep learning-powered NLU engine, for interactive voice conversations, making it possible for users to get medication reminders, track symptoms, and receive personalized wellness advice.
AI Models Used:
- Amazon Comprehend Medical (NLP)
- AWS HealthLake ML Models
- Lex Bot (based on Deep Neural Networks for NLU)
Babylon provides an AI-powered health assistant that can check symptoms, offer health assessments, and even schedule appointments with doctors.
Babylon’s AI Triage Assistant uses a hybrid model combining Bayesian inference and machine learning to simulate a doctor’s diagnostic reasoning. It’s built upon a vast medical knowledge graph using SNOMED CT ontology, allowing the assistant to cross-reference user-reported symptoms with thousands of clinical pathways. The AI system uses probabilistic models to evaluate potential diagnoses, adapting its logic based on symptom evolution and context. Babylon has also integrated deep reinforcement learning to optimize decision-making flows and improve clinical accuracy over time. The app interfaces via a chatbot and is capable of escalating users to human doctors when needed.
AI Model Used:
- Bayesian Diagnostic Model
- Deep Reinforcement Learning for decision optimization
- Rule-based systems + ML ensemble
The Microsoft Azure Health Bot is a highly customizable platform for building healthcare-compliant AI assistants. It’s used by hospitals, insurance providers, and public health organizations globally.
Microsoft’s Azure Health Bot is built on top of Microsoft Cognitive Services and incorporates components like Language Understanding Intelligent Service (LUIS) and Text Analytics API. It supports healthcare-specific capabilities such as symptom checking, triage guidance, and integration with FHIR-based medical data. The Health Bot also uses rule-based medical logic trees combined with transformer-based NLP models for intent detection, contextual analysis, and guided patient interaction. It complies with HIPAA standards and can be further enhanced using Azure OpenAI Service, enabling GPT-style conversational interfaces for clinical and administrative use cases.
AI Model Used:
- Language Understanding Intelligent Service (LUIS)
- Transformer-based intent classification models
- Option to integrate OpenAI GPT models via Azure OpenAI Service
Looking to integrate AI-driven virtual assistants into your medical service? Partner with Sunrise Technologies to create intelligent, compliant solutions.
Technology Used in AI Health Assistant Apps
AI health assistant apps are revolutionizing the way patients access care, communicate with providers, and manage wellness, all powered by an ecosystem of advanced technologies working seamlessly behind the scenes. Let’s break down the core components that make these apps smart, responsive, and personalized.
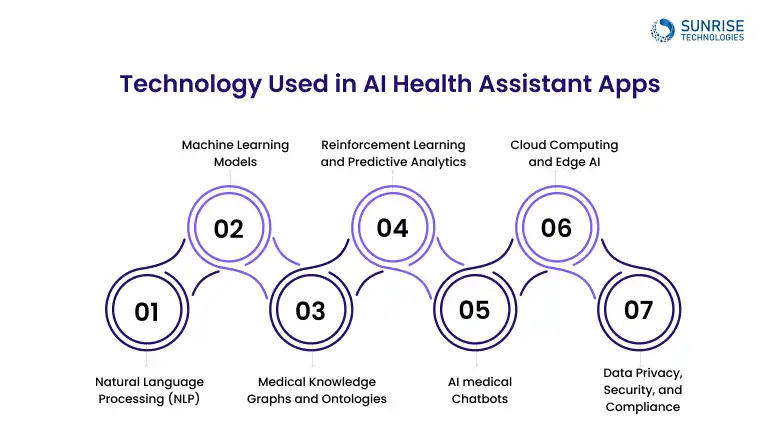
One of the most critical technologies enabling human-like conversations in these apps is natural language processing in healthcare.
- NLP models help the app understand user queries, extract medical symptoms, and translate everyday language into clinical terminology.
- Advanced tools like spaCy, BERT, and MedPaLM are commonly used in AI health assistant development to make dialogue more context-aware and medically relevant.
- NLP also enables multilingual support and personalized engagement.
Machine learning in healthcare apps are pivotal in providing diagnostic insights by recognizing patterns within health data. AI health assistant apps rely on a variety of supervised, unsupervised, and reinforcement learning models to process and evaluate health information.
- Classification algorithms such as Logistic Regression, SVM (Support Vector Machines), and Deep Neural Networks (DNNs) classify symptoms into probable diagnoses.
- Random Forest and XGBoost, commonly employed in medical diagnostic tools, enhance predictive accuracy by analyzing vast amounts of health data and generating robust decision boundaries.
- These ML models evolve as they ingest more patient data and refine their predictions, utilizing ensemble learning techniques to improve overall diagnostic capabilities.
For accurate clinical reasoning, AI systems rely on structured medical databases like SNOMED CT, ICD-10, and UMLS.
- These medical ontologies allow apps to recognize relationships between symptoms, diseases, drugs, and treatments.
- Ontologies such as SNOMED CT, ICD-10, and UMLS offer a structured framework to represent knowledge. For example, SNOMED CT provides standardized terms for clinical findings, procedures, and observations, while ICD-10 codes diseases and conditions.
- A medical knowledge graph in AI health apps enables semantic understanding and better decision-making.
To continuously improve and adapt to user behavior, many AI health apps use reinforcement learning in digital healthcare.
- The app observes user feedback and outcomes, learning to adjust its questioning strategy for better triage over time.
- Predictive analytics is used to anticipate potential health risks based on historical data and lifestyle inputs.
Most AI health assistants are delivered through AI-powered healthcare chatbots.
- These bots use a combination of dialog management, entity recognition, and intent classification to provide real-time health advice.
- Entity recognition, utilizing techniques like CRF (Conditional Random Fields) or NER, ensures that the chatbot correctly identifies medical entities from the user’s input, such as symptoms or conditions, which is then cross-referenced with clinical pathways.
- Speech-to-text APIs and voice assistants (e.g., Google Assistant, Alexa) are integrated into many apps for hands-free health assessments, enabling users to report symptoms and receive guidance via voice.s.
Scalability is key in digital health. That’s why cloud computing in AI health apps plays a crucial role.
- Cloud platforms like AWS HealthLake, Microsoft Azure HealthBot, or Google Cloud Healthcare API enable data storage, compute power, and secure model deployment.
- Some apps use Edge AI for real-time symptom analysis on smartphones, ensuring faster response and offline capability.
Handling sensitive health data demands top-notch security. That’s where HIPAA-compliant AI health assistant apps stand out.
- These apps follow strict data protection standards using end-to-end encryption, role-based access, and data anonymization.
- Role-based access control (RBAC) ensures that only authorized personnel can access certain levels of patient data, while two-factor authentication (2FA) and biometric security further safeguard user accounts.
- Compliance with GDPR, HIPAA, and HL7 FHIR ensures user trust and regulatory approval.
From startups to healthcare providers, we build intelligent, scalable solutions tailored to your needs.
Future Trends in AI Health Assistant Apps
The future of AI health assistant apps is poised to be smarter, more personalized, and deeply integrated with advanced healthcare infrastructure. With rapid developments in AI, wearable tech, and digital therapeutics, these virtual assistants will evolve into proactive healthcare companions. Let’s explore the exciting future trends shaping the next generation of these intelligent health solutions.
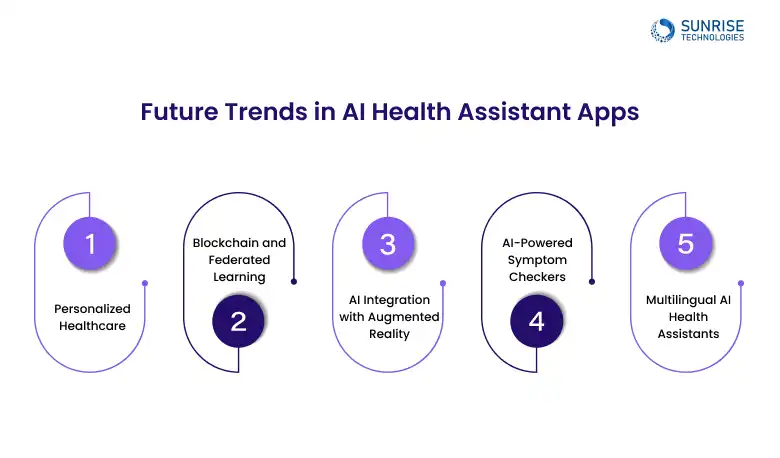
AI health assistant apps for personalized healthcare will soon offer hyper-personalized care by using genomics, EHRs, and lifestyle data. Powered by Graph Neural Networks and multimodal learning, they’ll adapt treatment suggestions to individual health profiles.
Future health bots will understand your mood through speech tone using sentiment analysis and SER (speech emotion recognition). This emotional intelligence will drive more empathetic and human-like responses.
AI apps will train on your data without ever storing it using federated learning. Blockchain will add an extra layer of trust and transparency for secure health data sharing.
AR-powered AI assistants will visually guide users through treatments or exercises using pose detection and real-time feedback. Great for rehab, self-care, and chronic condition management.
Next-gen symptom checkers will analyze contextual signals like weather, location, and ongoing outbreaks using contextual NLP and geo-tagged health data. This helps deliver more accurate and situational health advice instantly.
Language won’t be a barrier anymore,transformer-based models with neural machine translation will help AI assistants communicate in 100+ languages. This ensures health access for non-native speakers and underserved communities.
Why Choose Sunrise Technologies for AI Health Assistant App Development
With a proven track record across both continents, Sunrise Technologies has helped startups, hospitals, and enterprises build HIPAA-compliant AI health apps that scale. Our local presence and global expertise make collaboration smooth and results-focused.
Innovation shouldn’t break the bank. Our flexible pricing and modular development ensure you get affordable, high-performance apps that meet regulatory standards and user expectations.
From medical image analysis and clinical decision support systems to chatbot development using NLP and predictive analytics, our team brings in-depth technical knowledge backed by years of healthcare experience.
We create cross-platform AI apps that integrate seamlessly with EHR/EMR systems, HL7, and FHIR protocols, ensuring your AI health assistant app is both interoperable and future-ready.
We follow industry best practices and build apps in line with HIPAA, GDPR, and other regulatory standards, making patient safety and data privacy top priorities.
Our agile approach, transparent communication, and post-launch support ensure your AI app evolves with user needs and technology trends.
The evolution of AI health assistant apps is rapidly transforming how individuals access, manage, and understand their healthcare. Backed by technologies like Bayesian inference, deep learning, medical ontologies, and reinforcement learning, AI health assistants for startups in the USA are becoming trusted partners in our daily well-being, and as healthcare shifts toward prevention, personalization, and precision, the integration of AI into mobile healthcare AI solutions in Australia will continue to play a pivotal role in shaping a smarter, healthier future in Australia, and other countries.
As a top AI app development company in USA and Australia, we specialize in crafting custom AI health assistant apps that are scalable, and secure but also truly user-focused. So, you’re a healthcare startup aiming to disrupt the industry or a large hospital system looking for affordable AI apps, we’ve got the experience and the tools to help you get there. Recognized as one of the best AI development companies for healthcare, we blend medical intelligence with the power of machine learning to help you stay ahead in today’s fast-moving digital health world.
Let’s transform the future of healthcare together, one intelligent, personalized, and accessible AI health assistant app at a time
You can build your own customised AI apps with white-label AI health assistant apps. Cut operational overhead by 40%.
- 24/7 Accessibility: Instant healthcare advice available anytime, anywhere.
- Cost-effective Solutions: Affordable AI-powered assistance for healthcare providers.
- Personalized Insights: Custom health recommendations based on individual user data.
- Early Detection: Identifies potential health issues before they become serious.
- Efficient Triage: Smart assessments that prioritize care needs effectively.
- Convenience: Streamlines healthcare processes and saves valuable time.
These apps use machine learning and NLP to understand symptoms and provide recommendations.
Yes, most use encryption and follow healthcare compliance standards like HIPAA.
Apps like Ada Health, Babylon, and Wysa offer free or low-cost services with high efficiency. And for the best affordable apps, team up with a top AI development services like Sunrise Technologies.
The cost of developing an AI health assistant app depends on various factors such as app complexity, features, integrations, and the platform used. On average, the development cost can range from $30,000 to $150,000 or more.
Sam is a chartered professional engineer with over 15 years of extensive experience in the software technology space. Over the years, Sam has held the position of Chief Technology Consultant for tech companies both in Australia and abroad before establishing his own software consulting firm in Sydney, Australia. In his current role, he manages a large team of developers and engineers across Australia and internationally, dedicated to delivering the best in software technology.
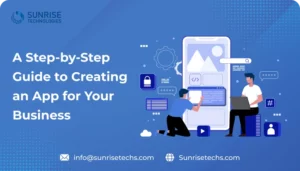
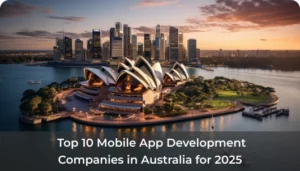
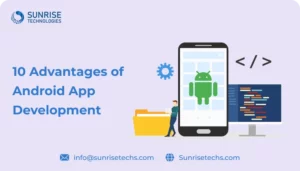
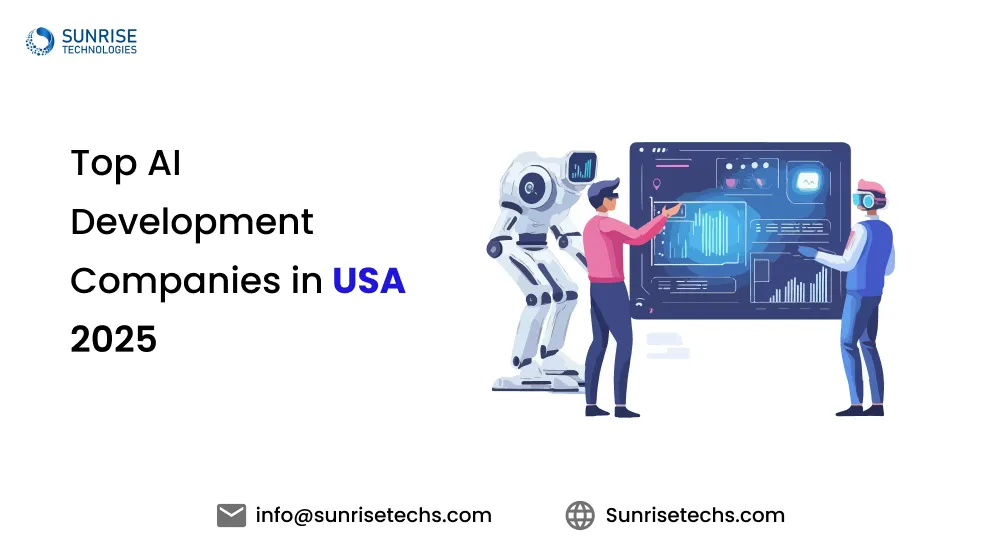
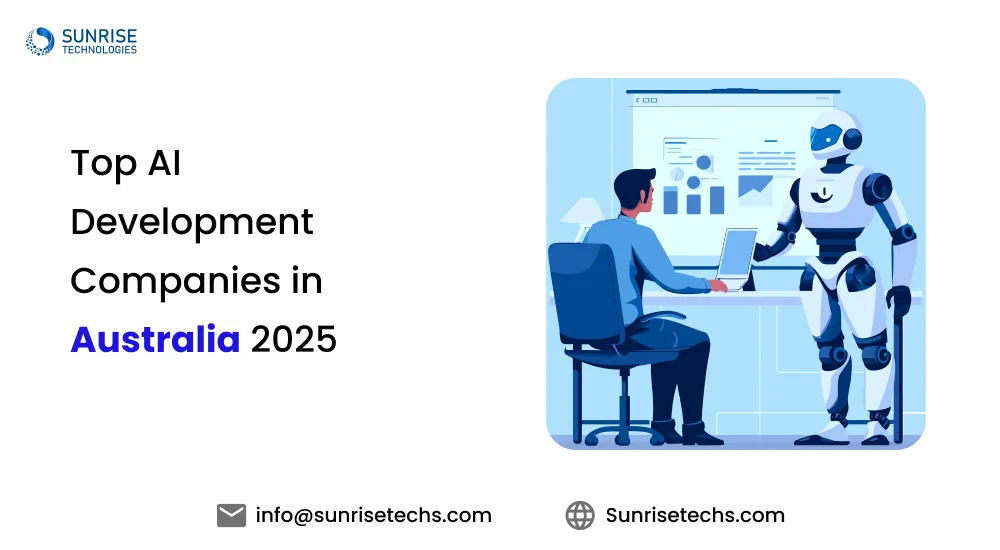
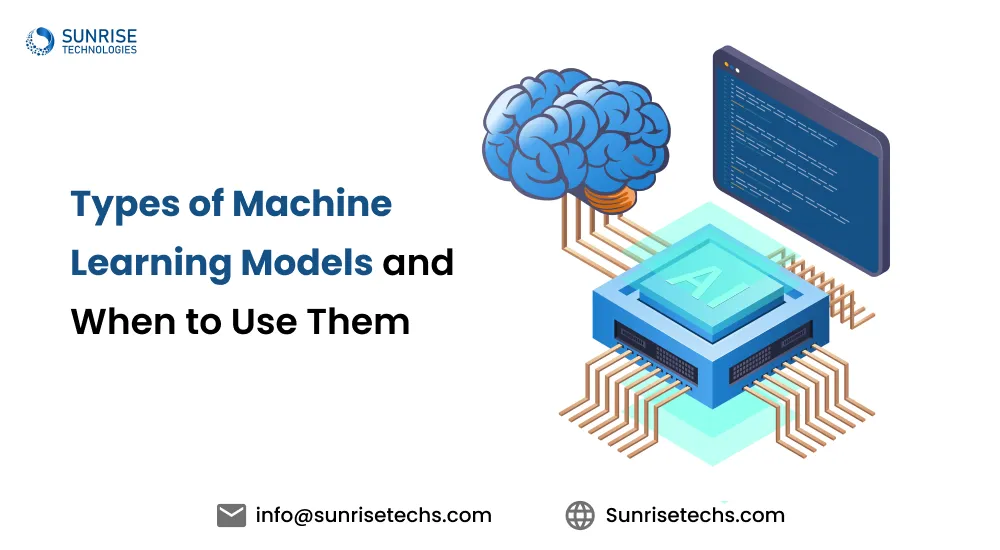
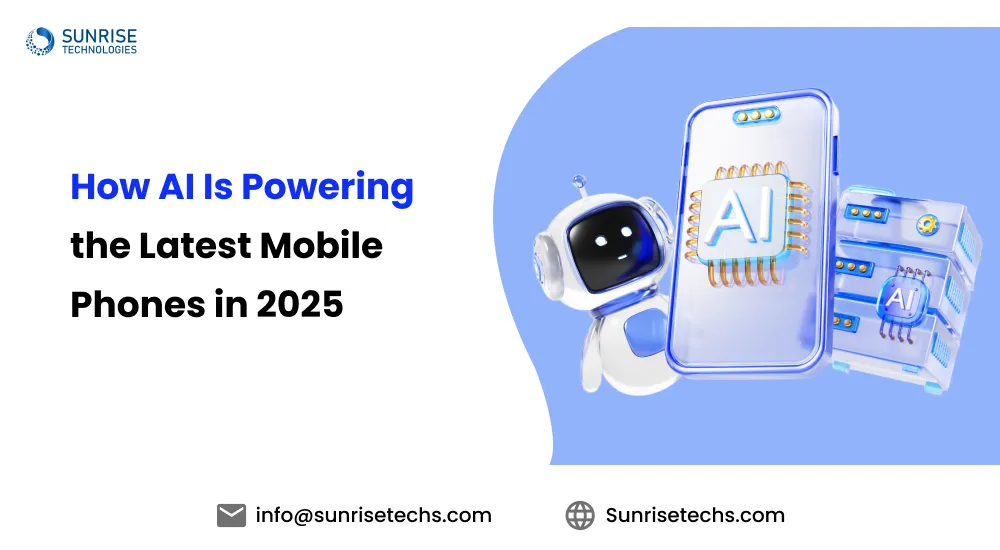
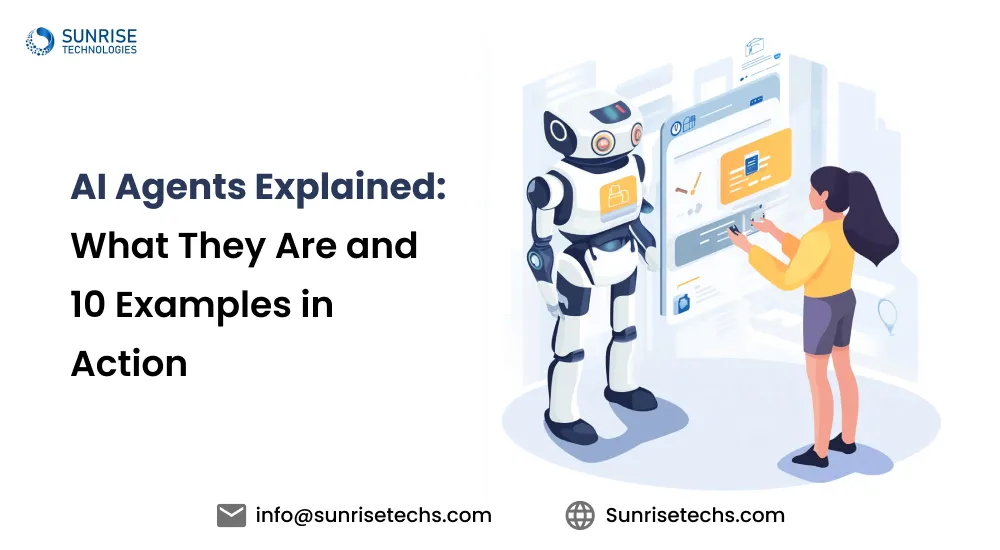